Dr. Ashar Ahmad
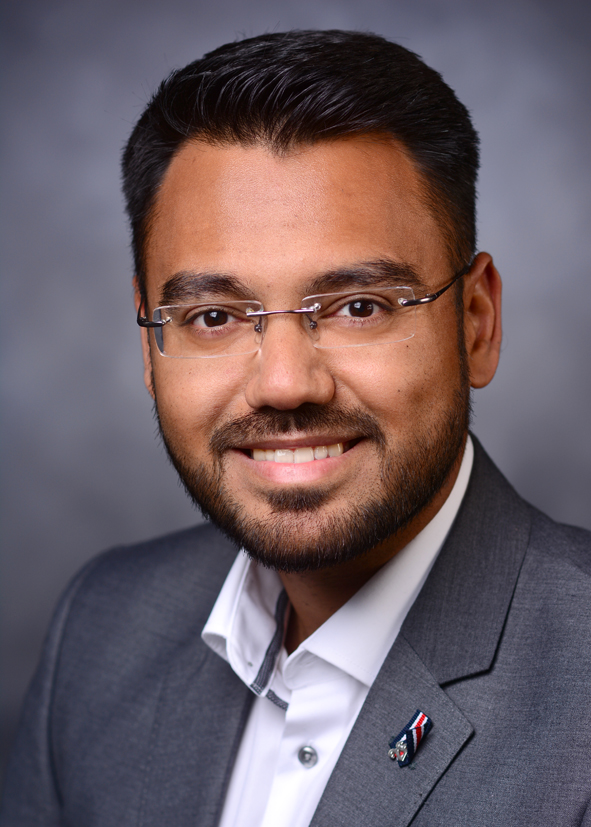
Title
Causal Machine Learning in Drug Development
Abstract
While Drug Development traditionally relies on controlled experiments to establish causality, for example in Pre-clinical animal PK/PD studies or RCTs in Clinical Development, there have been rapid advances in Causal Inference methodologies which have enabled the generation of causal evidence from Observational and Real World Data (RWD) sources at a population level.
Causal Machine Learning enables valid inferences on individualized treatment effects which could be potentially predictive in unseen patient cohorts, thus paving the way towards personalized treatment regiments and enrichment strategies in Clinical Development.
In this talk, I give an example of a use-case which is relevant to both Clinical and Commercial development of drugs - synthetic and external control arms which are being used either as comparator arms in Clinical Development or as head-to-head effectiveness comparisons with other medications in Commercial Development.
About Dr. Ashar Ahmad
Ashar Ahmad has a multidisciplinary background with a Chemical Engineering bachelor’s degree from IIT Bombay followed by Master’s degree in Computer Science specializing in Modelling and Simulation. Between 2014 and 2018 he worked at b-it in Prof. Dr. Holger Fröhlich’s lab on Statistical Machine Learning methodologies and contributing to translational projects at the University Medical Centre in Bonn.
After receiving his PhD, he joined UCB Pharma as a Post Doctoral Scientist working in the Translational Medicine department. Since 2021, he has been working as an Associate Director at Grünenthal GmbH in Aachen leading a team of Data Scientists and driving several high impact use-cases across Global R&D and Global Commercial business units.