SAFECAR-ML: Artificial Intelligence speeds up vehicle development
New machine learning methods are enabling better understanding and processing of crash test development data. The SAFECAR-ML project is developing an automated solution for documenting virtual crash tests based on artificial intelligence (AI). The aim is to simplify and enhance the efficiency of the design process.
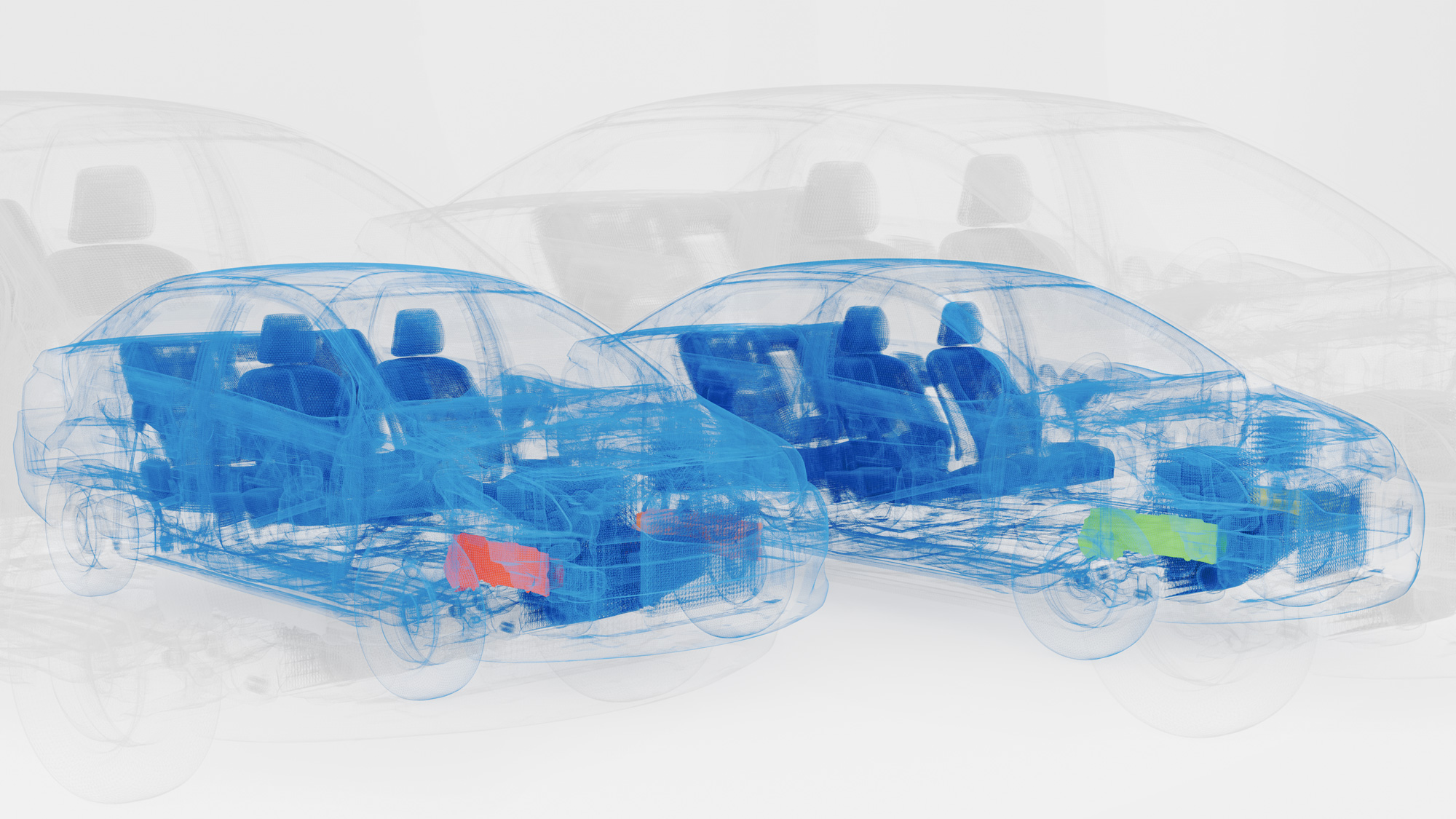
SANKT AUGUSTIN – Shattering glass, twisted metal, and flying debris are rare sights in today’s automotive crash tests, as most crash simulations are performed digitally. However, documenting every modification in the design of virtual crash vehicle models is crucial for development engineers. This is a tedious and expensive process. The goal of the new project SAFECAR-ML is to simplify this work. By combining novel artificial intelligence (AI) methods with technical knowledge from vehicle development, the project partners from research and the automotive industry aim to standardize data processing for documenting virtual crash tests.
Machine Learning (ML) makes it possible to interpret the engineers’ free-text inputs semantically. The unstructured data obtained in this way must be standardized and, for instance, linked to virtual vehicle data for ML processing. The software developed in the project will then automatically derive next steps and recommend actions.
“Changes to the virtual vehicle model can be recorded in natural language and automatically processed – similar to what large language models such as GPT-4 can already do. What is new is the integration of multimodal engineering data with ML. This approach has great potential for optimizing development processes through computer-aided engineering,” says Dr. Daniela Steffes-lai, a researcher in SCAI’s Numerical Data-Driven Prediction business unit. The goal of the project is to make vehicle development faster and more cost-effective overall.

Fraunhofer SCAI is contributing its extensive experience in ML and the comparative analysis of simulation results to the project. Its goal is to develop a formal description of technical knowledge for product development. Cooperation with the automotive industry opens the door for further applications in computer-aided engineering.
SCALE GmbH in Ingolstadt is the project partner working with Fraunhofer SCAI. The car manufacturers AUDI, Volkswagen, and Porsche are associated partners.
The German Federal Ministry of Education and Research (BMBF) is funding the project from September 2024 until February 2027 as part of the “Erforschung, Entwicklung und Nutzung von Methoden der Künstlichen Intelligenz in kleinen und mittleren Unternehmen (KI4KMU)” program. The total amount of funding is 670,000 euros.
Contact:
Dr. Daniela Steffes-lai
Business Area Numerical Data-Driven Prediction
Fraunhofer Institute for Algorithms and Scientific Computing SCAI
Schloss Birlinghoven 1, 53757 Sankt Augustin
www.scai.fraunhofer.de/en/ndv