Dr. Niklas Blomberg
Innovative Health Initiative (IHI JU)
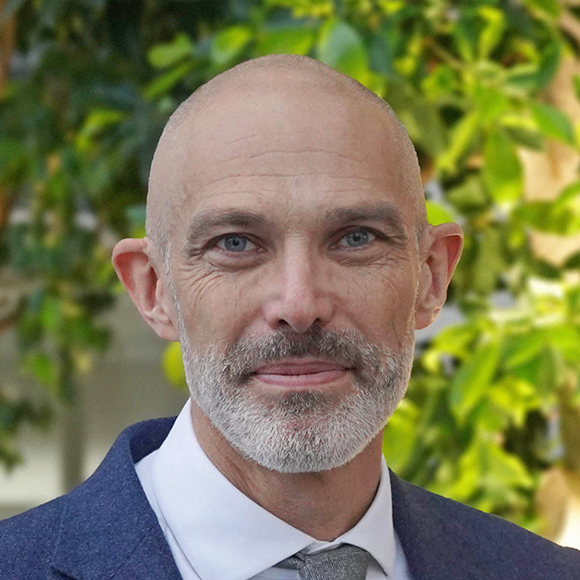
Title
IHI and the expectations of IHI towards the EHDS
About Dr. Niklas Blomberg
Dr. Niklas Blomberg joined IHI as Executive Director in January 2024. He brings to the role extensive experience in both research and leadership roles in the life sciences.
A Swedish national, he has a bachelor’s degree in chemistry from the University of Gothenburg, and a PhD in structural biology and bioinformatics from the European Molecular Biology Laboratory (EMBL) in Germany.
He worked as a research scientist for AstraZeneca in Sweden for 14 years, taking on increasingly senior roles in the company, and leading the establishment of a team for data driven drug discovery in the respiratory and inflammation fields. During this time, he was industry co-lead of Open PHACTS, a project funded by the Innovative Medicines Initiative (IMI), the forerunner to IHI.
In 2013, he joined the fledgling research infrastructure ELIXIR. As its founding director, he oversaw the final negotiations between the member states to formally establish and launch ELIXIR. Under his leadership, the organisation built up its operational and project management capacity, grew to include 23 member states, and secured funding from both the member states and an extensive portfolio of EU-funded projects, including projects from IMI2, the European Open Science Cloud and the European Genomic Data Infrastructure.